Flow Networks
We are interested in systems that can be modeled as flow networks, such as the animal or plant vasculature but also the power grid or experimental microfluidics. We try to understand how to change these networks to achieve different functionality or how nonlinearities can lead to complex phenomena.
A flow network made out of valves that have a region of negative differential resistance can lead to self-sustained traveling waves such as the ones presented in the video. If you want to know the details check our paper!
We are preparing experiments to probe the complex phenomena emerging in these systems, coming soon!
Flow networks can be modified to display different functionalities. One way to do this is through the minimization of a cost function, it turns out that we can control the topography of this landscape and this also motivated our work in machine learning (see below). Finally, we also study how the animal vasculature changes to optimize nutrient distribution, you can check out our preprint here.
Theory of Machine Learning
Inspired by our work on energy landscapes in the context of flow networks, we started to explore the possibility of changing the topography of the loss function landscape in machine learning to improve training. We realized we could do it in a very intuitive way, weighting more the contribution of each class, and cycling over all of them. It turns out that this controls the depth and width of the local minima, improving training for different problems. You can read our paper which was awarded a long talk at ICML2021, in case you want to watch it, it is here:
We are also interested in other problems that relate machine learning with how humans learn, check our paper about catastrophic forgetting or find out more below!
Applied Machine Learning
Machine learning is a great tool to tackle problems in other disciplines, and a great way to collaborate with colleagues from other fields. Right now we are collaborating with experimentalists, social and complex systems scientists, biologists and a psychologist. These are some projects that we are working on:
- Using graph neural networks to learn the interactions in systems of active particles. We use particle trajectories to extract the forces controlling its dynamics. Check out our preprint here. We are currently collaborating with experimentalists to apply these techniques to systems of living cells.
- We study social interactions in networks of high school students, it was interesting to measure how selfishness and intermediate contacts affect our chances to make a new friend (or enemy!). This is our preprint.
- We are collaborating in a project that addresses how children learn math, and how some knowledge transfer/interfere with others. Coming soon!
Stat Mech and Hard Condensed Matter
During my PhD, we studied models that coupled Ising spins to elastic membranes to understand rippling in 2D materials such as graphene. We also studied how thermal fluctuations affected the buckling of thin membranes. Finally, we studied the chaotic oscillations exhibited by semiconductor superlatices, and I even collaborated on a project that proposed how to use quantum dots to create a heat diode. I do not actively pursue these research lines at this time, but I am always happy to talk about them!
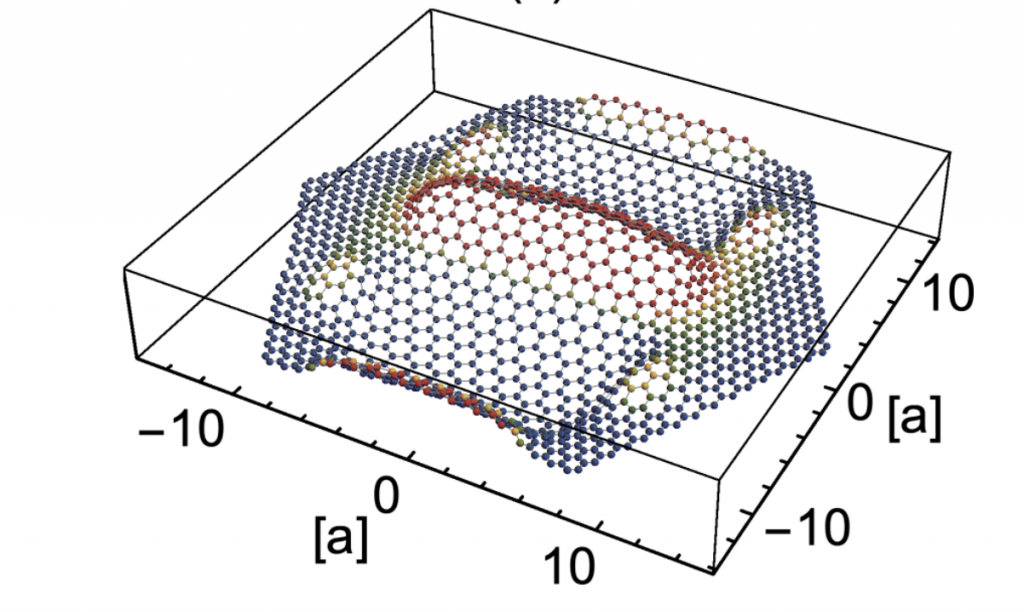
Publications
For an updated and complete list of my publications please visit my Google Scholar profile. If you do not have access to one of my manuscripts, please send me an email and I will be very happy to send it to you!